MSc thesis project proposal
[2024] Stochastic-resonance front-end for the acquisition of high-density cortical signals
In order to better understand the brain and better treat brain disorders, it needs to be neuromodulated by means of ‘brain-like’ waveforms1. Moreover, these waveforms need to be applied in a smart way, based on feedback and in a closed-loop fashion. This requires sensing technology, not only for reading the electro-chemical signaling of the brain itself but also of other physiological parameters. Additionally, this feedback needs to be self-learning so it can learn to recognize the (personal) brain activity and connectivity characteristics that characterize a symptom and the intensity of a symptom. It then selects an optimal stimulation design to normalize the symptom by increasing or decreasing connectivity to change the network structure. Finally, it can predict symptoms to prevent relapses of chronic disease states1.
The above needs are still far away from the state of the art. State-of-the-art neuromodulation is almost exclusively done using tonic rectangular pulses, at a single stimulation site, often not based on any form of feedback from the brain itself, and never self-learning. State-of-the-art technology for neural recording is not able to record the infraslow waves that modulate and thereby synchronize the more local brain activity, and, as it is either acquired from passive electrode arrays or from CMOS-based probes, is not able to reveal the brain’s small-world emergent network behavior2.
Innovative technology for both neuroscience (leading to a better understanding of the brain) and neuromodulation (leading to better treatment of brain disorders) should thus: 1. cover large parts of the brain for recording (reading) and stimulation (writing) and thus make use of flexible, stretchable arrays; 2. be minimally invasive; 3. excite or inhibit multiple neurons in various regions of the brain accurately (viz. with high spatiotemporal resolution), 4. with precisely controlled degrees of synchronicity amongst recorded or stimulated neural elements, 5. with more ‘brain-like’ stimulation patterns, such as noise, burst, infraslow waves, preferably using neuromorphic devices and self-learning interfaces (see figure), 6. by means of electrical, optogenetic, or other (e.g. ultrasound) neuromodulation (see figure); 7. record from, and stimulate, larger populations of neurons or assemblies than was hitherto possible; 8. do so in a ‘brain-like’ fashion that reduces data, but preserves information for self-learning and closed-loop control; and 9. last ideally forever, and thus be adaptive, upgradable, biocompatible, and biostable.
Assignment
In this MSc thesis project, we will be investigating the use of stochastic resonance and infra-slow waves to acquire cortical signals with unprecedented resolution, both in the spatial domain and in the magnitude domain. The resulting binary signals will be neuromorphically coded and eventually allow for ultra-low-power circuit implementation.
The MSc thesis activities will involve the following: 1. Literature study on neural / cortical recording; 2. Literature study on stochastic resonance; 3. System-level simulations of a cortical signal acquisition front-end employing stochastic resonance and neuromorphic coding of signals; 4. Hardware design of the front-end electronics using standard circuit building blocks; 4. Neuromorphic signal decoding using a suitable software platform (e.g., FPGA); If time permits, testing the front-end in vitro either in house or with our neuroscientific partners.
Requirements
We are looking for an MSc student in either Biomedical Engineering or Electrical Engineering, with good knowledge of either Matlab/Simulink or of high-level analog behavioral modeling (AHDL). Knowledge of analog and mixed-signal electronics and circuit simulation tools is not a must but a pre.
This is a scientific technology project that is unique in its kind and therefore extremely well suited for MSc students that might be interested in doing a PhD project directly after their MSc studies.
Contact
prof.dr.ir. Wouter Serdijn
Bioelectronics Group
Department of Microelectronics
Last modified: 2024-03-08
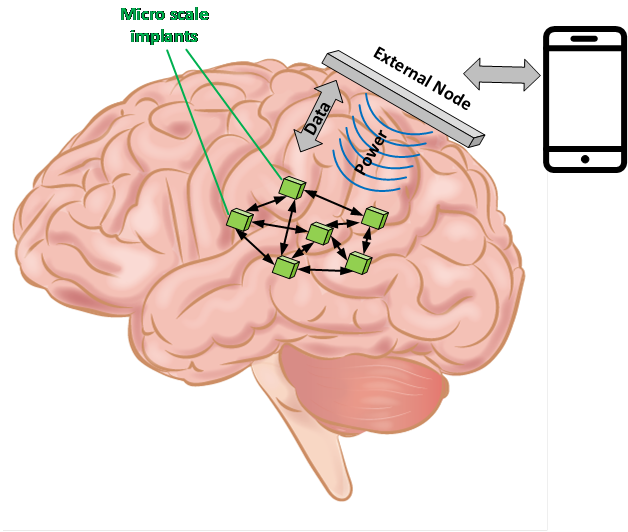